AI Learns to Create Detailed Maps Through Minecraft Exploration
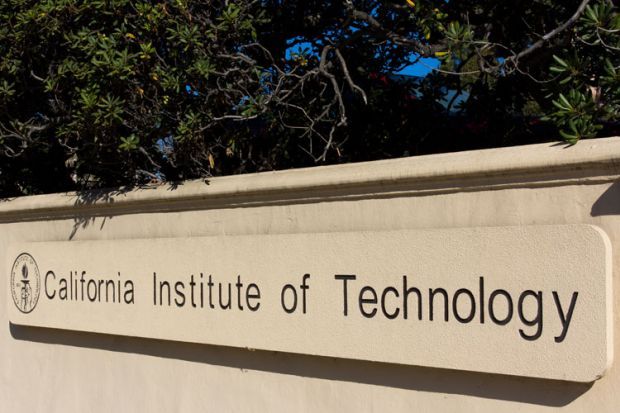
Imagine you are in the middle of an unknown town. Even if your surroundings are initially unfamiliar, you can explore around and eventually create a mental map of your environment—where the buildings, streets, signs, and so on are in relation to one another. This ability to construct spatial maps in the brain is the basis of even more advanced types of cognition in humans: For example, it is theorized that language is encoded in a map-like structure in the brain.
For all that cutting-edge artificial intelligence and neural networks can do, they cannot build maps out of nothing.
“There’s this sense that even state-of-the-art AI models are still not truly intelligent,” says Matt Thomson, assistant professor of computational biology and Heritage Medical Research Institute Investigator. “They don’t problem-solve like we do; they can’t prove unproven math results or generate new ideas. We think it’s because they can’t navigate in conceptual space; solving complex problems is like moving through a space of concepts, like navigating. AIs are doing more like rote memorization—you give it an input, and it gives you a response. But it’s not able to synthesize disparate ideas.”
A new paper from the Thomson lab finds that neural networks can be designed to build spatial maps using a type of algorithm called predictive coding. The paper appears in the journal Nature Machine Intelligence on July 18.
Led by graduate student James Gornet, the two built environments within the game Minecraft, incorporating complex elements like trees, rivers, and caves. They recorded videos of a player traversing the area randomly and used the video to train a neural network equipped with a predictive coding algorithm. They found that the neural network is able to learn how objects within the Minecraft world are organized relative to one another and was able to “predict” what environments were coming up while moving around the space. What’s more, the team “opened up” the neural network (the coding equivalent of “checking under the hood”) and saw that representations of the various objects were stored spatially with respect to one another—in other words, they saw a map of the Minecraft environment stored within the neural network.
Neural networks can navigate maps that have been given to them by human designers, such as a self-driving car using GPS, but this is the first time a neural network has been shown to create its own map. This ability to spatially store and organize information could ultimately help neural networks get “smarter,” enabling them to solve truly complex problems like humans can.
Gornet is a student in the Department of Computational and Neural Systems (CNS) at Caltech, which spans neuroscience, machine learning, math, statistics, and biology.
“The CNS program really gave James a place to do unique work that wouldn’t be possible anywhere else,” says Thomson. “We’re taking a bioinspired approach to machine learning that allows us to reverse-engineer properties of the brain in artificial neural networks, and we’re hoping to learn about the brain in turn. We have a very receptive community for this kind of work here at Caltech.”
The paper is titled “Automated construction of cognitive maps with visual predictive coding.” Gornet is the paper’s first author. Funding was provided by The David and Lucile Packard Foundation, the Tianqiao and Chrissy Chen Institute for Neuroscience at Caltech, the Heritage Medical Research Institute, and the Chan Zuckerberg Initiative. Matt Thomson is an affiliated faculty member with the Tianqiao and Chrissy Chen Institute for Neuroscience at Caltech.